The Journey to AI Maturity: Navigating the Path to Transformative Business Success
- Asgeir Ingason
- Nov 27, 2023
- 7 min read
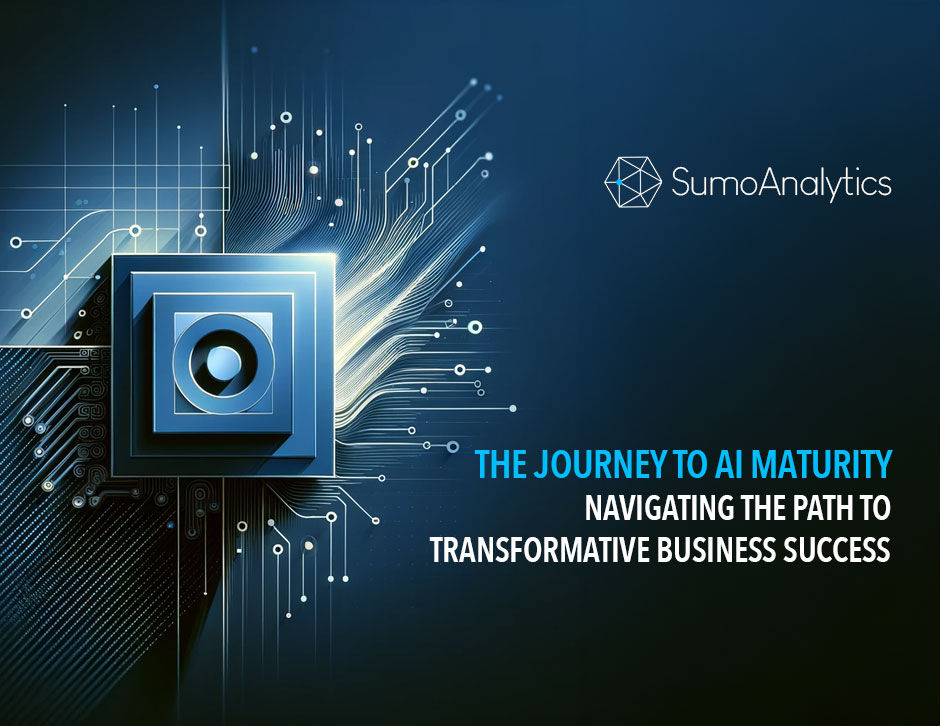
Artificial Intelligence (AI) maturity in companies signifies the extent to which AI is embedded in their operations and strategies. It's a critical measure in today's digital era, where AI's role ranges from automating tasks to driving innovation and growth. The journey to AI maturity involves not just the adoption of technology but a transformation in organizational culture and processes. This brief article explores the various stages of AI maturity, highlighting the top priorities at each level, the common reasons for the failure of AI projects, and essential do’s and don’ts, providing a clear roadmap for businesses aiming to leverage AI effectively.
The AI Maturity Spectrum
In the journey towards AI maturity, companies pass through distinct stages, each with its unique characteristics and challenges. Understanding these stages helps in aligning AI initiatives with organizational capacities and goals.
1. Initial Exploration
Characteristics: Organizations at this stage are beginning to recognize the potential of AI. Interest is sparked, but practical experience with AI is limited.
Challenges: A major challenge is overcoming initial skepticism and building a basic understanding of AI capabilities and limitations. Decision-makers often struggle with where to start and how to align AI with existing business processes.
2. Experimentation and Pilot Projects
Characteristics: Companies start small-scale AI experiments, typically in the form of pilot projects. This stage is about testing the waters, learning from practical experience, and understanding the implications of AI for the business.
Challenges: The primary challenge is selecting the right pilot projects that can provide meaningful insights without overwhelming resources. Another hurdle is managing expectations, as early experiments may not yield immediate or significant results.
3. Operational Integration
Characteristics: Successful pilot projects lead to the integration of AI into operational processes. AI begins to play a more significant role, enhancing efficiency, and contributing to decision-making.
Challenges: Integrating AI into existing systems and workflows can be complex, requiring not just technical adjustments but also cultural shifts within the organization. Ensuring that employees are on board and adequately trained to work alongside AI systems is crucial.
4. Strategic Adoption
Characteristics: AI is no longer seen as just a tool but as a strategic ally. It is deeply embedded in the company’s strategy, driving business decisions, innovation, and competitive advantage.
Challenges: The challenge at this level is to maintain momentum in AI adoption, continuously innovate, and avoid stagnation. Ensuring that AI initiatives remain aligned with the evolving business strategy and market conditions is critical.
5. Transformation and Innovation
Characteristics: At the peak of AI maturity, companies are not just using AI to improve existing processes but are leveraging it to drive major business transformations and create new business models.
Challenges: The key challenge is sustaining a culture of innovation and continuous improvement. Companies must also navigate ethical considerations and societal impacts of AI, ensuring responsible and transparent use of technology.
Understanding these stages helps companies assess where they stand in their AI journey and what they need to do to progress to the next level. It’s a roadmap that guides organizations from initial curiosity about AI to its full-scale adoption as a core driver of business transformation and innovation.
Strategic Priorities and Focus Areas
After understanding the different stages of AI maturity, it’s crucial to identify strategic priorities and focus areas for each stage. These priorities help organizations effectively navigate the challenges and capitalize on the opportunities at each level of their AI journey.
1. Initial Exploration
Priority: Building AI Awareness and Understanding
Focus on educating leadership and employees about AI's potential and limitations.
Conduct industry-specific research to understand how AI is being used by competitors and in the sector.
Initiate discussions about how AI could address current business challenges.
Priority: Laying the Groundwork for AI Adoption
Assess the current IT infrastructure's readiness for AI integration.
Begin cultivating a data-driven culture, emphasizing the importance of quality data for AI success.
2. Experimentation and Pilot Projects
Priority: Selecting and Managing Pilot Projects
Choose pilot projects that are aligned with business goals and have measurable outcomes.
Focus on quick wins that demonstrate AI's potential to stakeholders.
Priority: Developing Internal AI Expertise
Start building or acquiring the necessary AI talent.
Select strategic partnerships with AI agencies, such as Sumo Analytics.
Encourage cross-functional collaboration to integrate diverse perspectives and expertise.
3. Operational Integration
Priority: Integrating AI into Business Processes
Identify processes that would benefit most from AI enhancement.
Identify quick wins - low-hanging-fruits - to rapitly deliver value with the initial projects.
Ensure smooth integration of AI tools into existing workflows.
Priority: Scaling AI Initiatives
Evaluate the success of pilot projects and plan for scaling successful initiatives.
Foster a culture of continuous improvement, where feedback is used to refine AI applications.
4. Strategic Adoption
Priority: Aligning AI with Business Strategy
Integrate AI into the core strategic planning process.
Use AI to drive innovation and competitive differentiation.
Priority: Advanced Skill Development
Invest in AI training for employees.
Encourage a culture of lifelong learning and adaptation to new technologies.
5. Transformation and Innovation
Priority: Driving Business Transformation with AI
Leverage AI to explore new business models and revenue streams.
Encourage bold and innovative uses of AI that can lead to market disruption.
Priority: Ethical AI Deployment and Governance
Develop strong governance models to ensure ethical use of AI.
Stay informed about evolving AI ethics and regulatory landscapes.
By focusing on these strategic priorities, organizations can effectively advance through the stages of AI maturity, leveraging AI not just as a technological tool but as a transformative force that drives business growth, innovation, and competitive advantage.
Why AI Projects Fail
Despite the potential and promise of AI, not all projects succeed. Understanding the common reasons for failure can help organizations avoid pitfalls and steer their AI initiatives towards success.
Lack of Clear Strategy and Objectives
Misalignment with Business Goals: Many AI projects fail because they are not aligned with the company's strategic objectives. Without a clear connection to business goals, AI initiatives can lack purpose and direction.
Unclear Objectives: Projects often falter when their goals are not clearly defined. Vague or shifting objectives make it difficult to measure success and can lead to scope creep.
Inadequate Data Infrastructure
Poor Data Quality: AI systems require high-quality, relevant data. Inaccuracies or incomplete data can lead to flawed insights and ineffective AI models.
Lack of Access to Data: Sometimes, even when quality data exists, it may be siloed within different parts of the organization, making it inaccessible for AI projects.
Resistance to Change
Organizational Culture: Resistance to change is a significant barrier. If the organizational culture does not support innovation and adaptation, AI projects can face internal resistance.
Lack of Employee Engagement: AI initiatives require active involvement from various departments. Lack of engagement from key stakeholders can hinder the progress and adoption of AI projects.
Insufficient Skills and Expertise
Talent Gap: The success of AI projects heavily depends on having the right talent with the necessary technical and business expertise.
Inadequate Training: Failure to provide sufficient training for employees to work effectively with new AI systems can lead to underutilization and inefficiencies.
Ethical and Governance Issues
Ethical Concerns: Neglecting ethical considerations, such as bias in AI algorithms, can lead to public backlash and regulatory issues.
Lack of Governance Frameworks: Without proper governance structures, AI projects can become ungovernable and risky, especially in terms of data privacy and security.
Unrealistic Expectations
Overhyping Capabilities: Overestimating what AI can achieve in the short term can lead to disappointment and perceived failure.
Lack of Patience for Long-Term Results: AI projects often require time to show results. Impatience and the demand for immediate returns can lead to premature abandonment of potentially successful initiatives.
Understanding and addressing these issues is crucial for the success of AI projects. By recognizing these challenges early on, organizations can take proactive steps to mitigate risks and set their AI initiatives on the path to success.
Do’s and Don’ts at Different Stages
Effective navigation through the stages of AI maturity requires not only understanding the right strategies but also being aware of the common pitfalls. Here are key do’s and don’ts for each stage of AI maturity:
1. Initial Exploration
Do:
Educate your team about AI's potential and limitations.
Start small and focus on understanding how AI can solve specific business problems.
Encourage a culture of curiosity and open-mindedness towards new technologies.
Don’t:
Rush into adopting AI without a clear understanding of its implications.
Underestimate the importance of a data-driven culture.
2. Experimentation and Pilot Projects
Do:
Select pilot projects that are manageable and have clear objectives.
Involve cross-functional teams to gain diverse perspectives.
Use pilot projects as learning opportunities, even if they don’t yield immediate results.
Don’t:
Ignore the importance of quality data.
Overlook the need to manage change and set realistic expectations.
3. Operational Integration
Do:
Integrate AI solutions into business processes where they can add the most value.
Focus on employee training and change management to ensure smooth adoption.
Regularly assess and refine AI applications based on feedback and results.
Don’t:
Neglect the scalability and interoperability of AI solutions.
Underestimate the importance of aligning AI initiatives with overall business strategy.
4. Strategic Adoption
Do:
Embed AI into your core business strategy.
Foster a culture of innovation and continuous learning.
Invest in advanced AI skills and training for your team.
Don’t:
Become complacent with initial successes.
Overlook ethical considerations and the need for robust AI governance.
5. Transformation and Innovation
Do:
Leverage AI to drive business transformation and explore new business models.
Stay abreast of the latest AI advancements and think long-term.
Prioritize ethical AI practices and maintain transparent governance.
Don’t:
Cease experimentation and risk-taking with AI.
Ignore the broader societal impacts of AI applications.
By adhering to these do’s and don’ts, organizations can effectively navigate the complexities of AI adoption at each stage, avoiding common pitfalls and steering their AI initiatives towards success and sustainability.
Conclusion
The journey towards AI maturity is a nuanced and multi-staged process that requires careful planning, execution, and adaptation. We've explored the various levels of AI maturity, each marked by its distinct characteristics and challenges. From the initial exploration where companies start to understand the potential of AI, to the stage of transformation and innovation where AI becomes a catalyst for business reinvention, each step in this journey is crucial.
Key priorities at each level include building AI awareness, managing pilot projects, integrating AI into operations, aligning AI with business strategy, and ultimately, using AI for transformational change. Understanding why AI projects fail — be it due to lack of clear objectives, inadequate data, resistance to change, skill gaps, or ethical issues — is essential for navigating this path successfully.
The do’s and don’ts highlighted for each stage serve as a practical guide for companies to avoid common pitfalls and leverage AI effectively. It's important to remember that AI maturity is not a static achievement but a continuous process of learning, adapting, and evolving. AI should be viewed as a journey, not a destination, where each step forward opens up new possibilities and challenges.
Final Thoughts
This exploration into AI maturity is just the beginning. Each organization's journey will be unique, influenced by its specific context, industry, and aspirations. We invite further discussion and queries from readers who are navigating their own paths towards AI maturity. Whether you are just starting out or well on your way, remember that the journey of AI is one of constant discovery, growth, and adaptation. Your experiences, insights, and lessons learned are invaluable contributions to the broader narrative of AI in the business world.
Sumo Analytics is a data science and AI laboratory, specializing in the realm of prediction science. We build and deploy advanced AI systems that elegantly marry human intelligence with the computational power of artificial intelligence, enabling our clients to achieve unparalleled performance.