HOW HOSPITALS ARE USING PREDICTIVE MODELS TO EASE EMERGENCY DEPARTMENT CONGESTION
- Asgeir Ingason
- Oct 5, 2021
- 4 min read
Updated: Jun 12, 2023
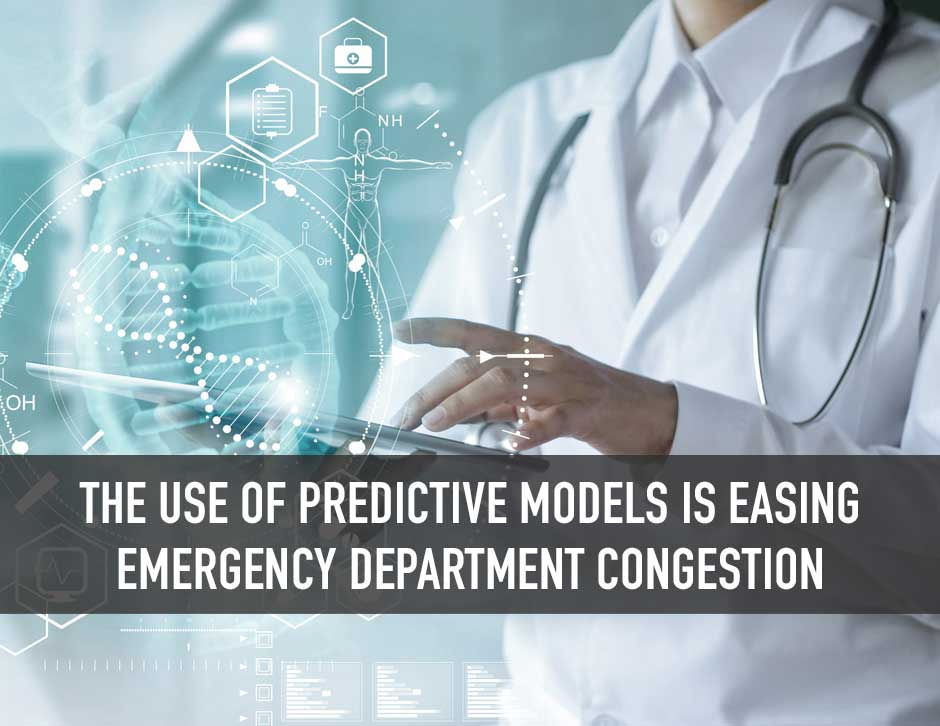
Healthcare institutions are increasingly adopting analytics capabilities in order to streamline their operations. Although we’re talking about patients, the same logic applies as with physical products; data science and advanced analytics can improve planning and decision making and increase efficiency in operations which will benefit the hospital as much as the patients. The main change is happening with the adoption of machine learning models and deep neural networks, where hospitals are utilising predictive analytics in their approach to solve prestisting operational challenges.
Crowding in emergency departments (EDs) is a challenge anywhere in the world and everybody who’s ever had to visit the ED knows it can be overwhelmingly crowded with long waiting times. Unless your Emergency Severity Index (ESI) is 1 or 2, which means that time is of essence to save your life, you’ll be waiting there for hours.
The first question to ask would be why there is congestion in the EDs. Is there simply a lack of staff to attend patients? Is the hospital too small for the size of the population in its service area? It’s probably both, but the problem is a bit bigger than that. When it comes to congestion within the EDs, the problem is mainly threefold.
Firstly, congestion in the ED is usually because of the inability to transition patients into inpatient units within the hospital efficiently. Boarding is a “patient who remains in the ED after the patient has been admitted or placed into observation status at the facility, but has not been transferred to an inpatient or observation unit” according to a definition by the American College of Emergency Physician. The problem with boarding inpatients is surprisingly common globally, where all seem to be dealing with the same causes.
Secondly, patients are faced with delays with their transfer because of understaffing. Obviously lack of staff can simply be just that. I.e. there isn’t enough staff due to lack of funding or whatever reason. However, this could also partly be a planning problem, and that’s precisely what hospitals are realising. How can shifts be organised with the right number of staff if it’s not known how many patients are coming?
Thirdly, one reason why patients are faced with delays with their transfer is because of lack of ward beds, and the problem could obviously be just that there aren’t enough beds. But this could also be a planning problem. How can beds and departments be organised if it’s not known for how many patients are expected and how long they are staying?
Here’s how hospitals are solving these persisting problems by adopting a data-driven approach:
Emergency Department Visits Forecasting
Just by having more accurate information about how many are visiting the ED and when, is already a big step. By adopting advanced forecasting methodologies and developing real-time analytics systems for decision support, hospitals are forecasting ED arrivals on a short and long time-horizon, using data from prehospital services.
Notification data from ambulances and police are being tremendously beneficial for 60 min forecasts. But historical data of ED visits is turning out to be extremely valuable for forecasting visits for the next 7 days. With advanced AI-driven forecast models, external variables such as weather and the level of tourist arrivals are adding to the accuracy. Even data about unemployment rate are being used to forecast long-term. The methods and technologies used for forecasting rely on advanced AI and machine learning, and are tremendously precise.
When armed with accurate forecasts about ED visits, all planning around staffing becomes more accurate as well. Whereas understaffing can be life threatening, overstaffing is simply expensive. This problem is being reduced significantly by hospitals and healthcare clinics around the world with the adoption of accurate AI-driven forecasts.
Patient Admission Prediction Modeling
ED congestion is commonly caused by the ineffectiveness of transitioning patients into inpatient units within the hospital in a timely manner. Hospitals are developing sophisticated prediction models driven by deep learning techniques that can accurately estimate the likelihood of patient admission.
These models are used as decision support tools and help reduce ED boarding and crowding by predicting ED patient admission using demographic and clinical information.
Predicting Inpatient Length Of Stay
In order to plan and organise bed allocation with proper lead time, it’s critical to estimate the patients length of stay (LoS). Major development in the use of artificial intelligence has allowed hospitals to predict LoS with surprisingly high accuracy.
By utilising data of vital parameters measurements from the ED, along with chemistry tests, diagnoses, medications, medical history and precious visits, hospitals are managing to create a clear picture of the inpatient population and their length of stay. These predictions allow hospital managers to improve their planning of required resources and the proceedings of assigning beds to patients.
xxx
Although hospitals have lagged behind in the adoption of AI-solutions, they are certainly catching up. Data-driven transformation is changing how they operate, where data science and advanced analytics are being adopted by hospitals and healthcare clinics worldwide in order to improve operations and increase efficiency.