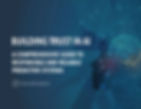
In an era where artificial intelligence (AI) is increasingly shaping our world, trust in these advanced systems has become a cornerstone issue for businesses, governments, and individuals alike. As AI systems grow more complex and integrated into our daily lives, fostering trust in their predictions and decisions is not just desirable—it's essential.
Trust, in this context, is the collective confidence that stakeholders place in an AI system's actions, predictions, and decisions. It's the belief that these AI systems will perform as expected, free from bias, and within the confines of regulatory and ethical norms. Yet, the question remains, how do we, as a society, instill such trust?
This is no trivial task. AI is a double-edged sword; it holds the potential to revolutionize industries, unlock new insights, and improve efficiencies, but it also carries risks, including those related to privacy, security, and bias.
Consider autonomous vehicles, an industry heavily reliant on AI. The transformative potential here is immense—reducing accidents, improving traffic flow, and providing unprecedented mobility options. But the technology can only reach its full potential when riders, other motorists, and pedestrians trust the AI making critical decisions at high speeds.
Similarly, in healthcare, AI could significantly improve diagnostics, personalized treatments, and predictive health monitoring. However, the sensitivity of health data and the critical nature of healthcare decisions necessitate a high degree of trust in the AI systems used.
At Sumo Analytics, we specialize in data science and AI research, particularly in prediction science. We understand the nuances of AI systems and the importance of trust in their successful implementation. This article aims to delve into the complexities of building trust in AI, exploring various dimensions such as data quality, model accuracy, robustness, stability, and velocity. We will also discuss the importance of compliance, security, humility, and governance in operational trust, along with ethical considerations like transparency, bias and fairness, and privacy implications.
As we navigate the path towards an AI-integrated future, understanding these facets and fostering trust in AI systems will be the key to unlocking the full potential of AI technologies across industries. This journey is not just about technological advancement—it's about aligning AI with our societal values and expectations.
Understanding AI Systems
Artificial Intelligence (AI) systems, at their core, are computational models designed to perform tasks traditionally requiring human intelligence. These tasks range from understanding natural language and recognizing patterns to making predictions and decisions. AI systems can learn from experience, adapt to new inputs, and perform complex tasks with varying degrees of autonomy.
The mechanics of AI involve several interconnected elements. It starts with data—large volumes of information used to train and refine the system. Then, using algorithms, AI systems identify patterns and structures within the data. These algorithms can range from simple rules to complex neural networks that mimic the functioning of a human brain. As these systems receive more data, their predictions and decisions improve over time, a concept known as machine learning.
Prediction science plays a significant role in AI. It involves using historical data to predict future outcomes. The precision and reliability of these predictions are fundamental to the effectiveness of AI systems. Prediction science is everywhere in AI, from predicting customer behavior in marketing to anticipating stock market trends in finance, forecasting patient health outcomes in healthcare, and estimating equipment failure in manufacturing.
At Sumo Analytics, we understand the power and potential of AI systems, particularly the aspect of prediction science. Our work involves harnessing this potential to help organizations make informed decisions. We leverage high-quality data, sophisticated algorithms, and robust models to deliver accurate predictions. These predictions guide businesses, helping them understand potential future scenarios, take proactive measures, and ultimately drive performance.
Our expertise also allows us to acknowledge and address the challenges associated with AI, including those related to trust. We recognize that for AI systems to be truly effective, they need to be trustworthy. This means they should not only be accurate and reliable but also transparent, fair, secure, and respectful of privacy. As we delve deeper into the dimensions of trusted AI in the subsequent chapters, we'll continually link back to how these dimensions are addressed in our work at Sumo Analytics. By doing so, we hope to provide practical insights into fostering trust in AI systems, bringing us one step closer to realizing the full potential of AI technologies.
Practical Dimensions of Trusted AI
Building trust in AI systems involves a combination of several practical dimensions, each contributing to the overall reliability and effectiveness of the system. These dimensions—data quality, model accuracy, robustness, stability, and velocity—are the foundations upon which trusted AI is built.
Data Quality
The adage "garbage in, garbage out" is particularly apt when discussing AI. The quality of the data used to train and refine an AI system directly influences its predictions and decisions. High-quality data is accurate, complete, diverse, and representative of the real-world scenarios the AI system will encounter. Poor quality data, on the other hand, can lead to inaccurate predictions, reinforce existing biases, or even cause the system to fail.
For example, an AI system trained on data with many missing values, inaccuracies, or unrepresentative samples may learn incorrect or biased patterns, affecting its accuracy and robustness. Therefore, ensuring data quality is a critical first step in building trust in AI systems.
Model Accuracy
The accuracy of an AI model—the degree to which its predictions match the actual outcomes—plays a significant role in fostering trust. If a model consistently provides accurate predictions, users are more likely to trust its outputs.
However, achieving model accuracy is a delicate balancing act. Overfitting and underfitting are two common pitfalls that can compromise accuracy. Overfitting occurs when a model learns the training data too well, to the point of including noise or anomalies, and performs poorly on new data. Underfitting, on the other hand, happens when the model fails to learn the underlying patterns in the data, resulting in inaccurate predictions both on the training data and new data.
By carefully tuning and testing AI models, we can avoid overfitting and underfitting, improving model accuracy and fostering trust in the system's outputs.
Robustness
Robustness in an AI system is its ability to handle new, unexpected, or outlier data and still maintain performance. In a world where data is continually changing, robustness is a crucial characteristic for maintaining the utility and trustworthiness of an AI system over time.
A robust AI system can effectively deal with shifts in the data landscape—new trends, different user behaviors, or changing market conditions—and still provide reliable outputs. This resilience in the face of change enhances users' confidence in the system, making it a critical factor in building trust.
Stability
Stability refers to the consistency of an AI system's outputs over time. A stable AI system delivers reliable, consistent results, building user confidence and fostering trust. If an AI system's predictions fluctuate wildly or change drastically with slight variations in input data, users may question its reliability and lose trust.
Ensuring stability involves rigorous testing and validation of AI models, along with careful monitoring and maintenance once the system is deployed.
Velocity
Velocity, or the speed at which an AI system can provide predictions, plays a crucial role in certain use cases. In scenarios where real-time or near-real-time decisions are required—such as fraud detection in finance or anomaly detection in cybersecurity—fast, accurate predictions are key to the system's effectiveness and trustworthiness.
However, speed should never come at the cost of accuracy or quality. A fast but inaccurate prediction can be as detrimental as a slow one, if not more. Balancing velocity with other dimensions of trusted AI is therefore crucial.
In sum, building trust in AI systems involves paying careful attention to these practical dimensions. By ensuring high data quality, model accuracy, robustness, stability, and appropriate velocity, we can create AI systems that users can confidently rely on. At Sumo Analytics, these dimensions form the bedrock of our AI development process, helping us deliver trustworthy, reliable AI solutions to our clients.
Operational Trust in AI: Compliance, Security, Humility, and Governance
As we delve into the operational aspects of fostering trust in AI systems, we see that it encompasses a range of considerations beyond the technical and practical dimensions. It involves meeting regulatory requirements, securing data and systems, acknowledging the limitations of AI, and establishing governance frameworks. Each of these factors plays a crucial role in building operational trust in AI systems.
Compliance
Compliance with relevant laws, regulations, and standards is a non-negotiable aspect of building trust in AI systems. This includes data protection laws such as the General Data Protection Regulation (GDPR) in the European Union, industry-specific regulations, and standards related to ethical AI use.
AI systems must be designed and operated in a manner that respects these regulatory requirements. Non-compliance can result in legal repercussions, financial penalties, and a loss of stakeholder trust. Furthermore, compliance should not be seen as a mere box-checking exercise but as a commitment to ethical, responsible AI use.
Security
Security is another key pillar of operational trust in AI. As AI systems often process sensitive data and make important decisions, they are attractive targets for cyberattacks. A security breach can compromise the integrity of the AI system, leading to erroneous predictions, privacy violations, and a loss of trust.
Securing AI systems involves safeguarding the data, protecting the models and algorithms, and ensuring the resilience of the system against attacks. This requires a robust cybersecurity strategy, regular vulnerability assessments, and a proactive approach to threat detection and response.
Humility
Humility in AI refers to the system's ability to recognize and communicate its own limitations. No AI system is infallible or capable of handling every possible scenario. A trustworthy AI system should be able to identify situations that fall outside its training or competence, and respond appropriately—whether that's asking for human intervention, declining to make a prediction, or indicating the level of uncertainty in its output.
This humility not only builds trust but also enhances the safety and reliability of AI systems. It ensures that decisions are not made blindly based on AI outputs but are considered in the context of the system's capabilities and confidence.
Governance
Finally, governance is the organizational structure and processes established to oversee and guide the use of AI. Effective AI governance involves clear policies, roles, and responsibilities, as well as oversight mechanisms to ensure the AI system is used ethically and responsibly.
Governance also includes processes for monitoring and managing the performance of the AI system, addressing issues and concerns, and maintaining transparency and accountability. An effective governance framework can help prevent misuse of AI, address potential issues proactively, and foster trust among stakeholders.
In conclusion, operational trust in AI involves a combination of compliance, security, humility, and governance. At Sumo Analytics, we understand the importance of these aspects and incorporate them into our AI development and deployment processes, furthering our commitment to building trustworthy, reliable AI systems.
Ethical Considerations for Trustworthy AI Systems
Building trust in AI systems is not just about the technical and operational aspects; it also involves addressing a range of ethical considerations. These considerations—transparency, bias and fairness, and privacy—are fundamental to aligning AI systems with our societal values and expectations.
Transparency
Transparency in AI systems refers to the ability to understand how the system works, makes its predictions, and arrives at decisions. It's about making the AI process clear and comprehensible to stakeholders, including users, regulators, and the public.
Opaque AI systems, often referred to as "black boxes," can make it difficult for stakeholders to trust the system's outputs. If people don't understand how a decision was made, they may question its validity, fairness, and reliability.
To foster trust, AI systems should be as transparent as possible. This involves clear documentation of the system's design and operation, explanations of its outputs, and openness about its limitations and uncertainties. Transparency also facilitates accountability, allowing stakeholders to hold the system (and its operators) accountable for its decisions.
Bias and Fairness
Bias in AI can occur when the system's predictions or decisions systematically favor certain groups or outcomes over others. This can happen due to biases in the training data, biased algorithms, or a lack of diversity in the AI development team.
Bias in AI can lead to unfair outcomes, such as discrimination or exclusion, and erode trust in the system. It's therefore crucial to ensure fairness in AI systems. This involves using diverse and representative training data, testing the system for biases, and taking corrective action if biases are found.
At Sumo Analytics, we take bias and fairness seriously. We use robust methods to detect and mitigate biases in our AI systems, and we're committed to promoting fairness and inclusivity in AI.
Privacy
Privacy is another critical ethical consideration for AI systems. As AI often involves processing personal or sensitive data, respecting user privacy is essential to building trust.
This involves complying with data protection laws, using anonymization or pseudonymization techniques, and only collecting and using data with the user's informed consent. It also involves being transparent about how the data is used and giving users control over their data.
Privacy breaches can have serious consequences, including legal penalties, reputational damage, and a loss of user trust. Therefore, privacy should be a top priority in the design and operation of AI systems.
In conclusion, ethical considerations are fundamental to building trust in AI systems. By ensuring transparency, addressing bias and promoting fairness, and respecting user privacy, we can align AI systems with our societal values and foster trust among stakeholders. At Sumo Analytics, we're committed to ethical AI use, and we embed these principles into our AI development and deployment processes.
Case Study: Trusted AI in Action
To illustrate how the principles of trusted AI can be applied in a real-world context, let's consider a recent project carried out by Sumo Analytics for a major healthcare provider.
The project involved developing an AI system to predict the likelihood of hospital readmissions within 30 days. This is a significant issue in healthcare, as high readmission rates can indicate lower quality of care and lead to higher costs. The goal was to use these predictions to identify high-risk patients and intervene earlier to prevent unnecessary readmissions.
Data Quality and Model Accuracy
The project began with an extensive data collection and cleansing process. We sourced data from a variety of hospital records, ensuring a diverse and representative sample. We also carried out rigorous data cleaning to deal with missing values and outliers, ensuring the data's quality.
For the AI model, we used a machine learning algorithm known for its accuracy and interpretability. We carefully tuned the model to avoid overfitting and underfitting, testing it on separate data to verify its accuracy.
Robustness, Stability, and Velocity
The AI system was designed to handle a wide range of patient data and to maintain its performance even as new data came in. It was also built to provide consistent predictions over time, contributing to its stability.
In terms of velocity, the system was capable of processing new patient data and updating its predictions in near-real-time, allowing healthcare providers to act quickly on its insights.
Compliance, Security, Humility, and Governance
Compliance with healthcare regulations, including data protection laws, was a top priority throughout the project. We also implemented robust security measures to protect the sensitive patient data the system was handling.
The AI system was designed to acknowledge its limitations. For example, it included a measure of uncertainty with its predictions and flagged cases that fell outside its training data for human review.
As for governance, the healthcare provider set up a steering committee to oversee the use of the AI system, establishing clear policies and responsibilities and ensuring ethical, responsible AI use.
Transparency, Bias and Fairness, and Privacy
Transparency was ensured through clear documentation and explanations of the AI system's workings and decisions. We also carried out bias testing and mitigation to promote fairness in the system's predictions.
Respecting patient privacy was paramount. We used anonymization techniques to protect patient identities and were transparent with patients about how their data would be used.
The project was a success, leading to a significant reduction in readmission rates and demonstrating the potential of trusted AI in healthcare. It serves as a prime example of how, by carefully addressing the dimensions of trusted AI, we can build AI systems that are not only effective but also trustworthy and aligned with our societal values.
Conclusion
In this rapidly evolving digital age, Artificial Intelligence has transformed from a novelty to a necessity. It has the potential to revolutionize sectors from healthcare to autonomous vehicles, and beyond. However, the crux of successfully harnessing this potential lies in one crucial aspect: trust.
We have journeyed through the key dimensions that contribute to establishing trust in AI systems: data quality, model accuracy, robustness, stability, and velocity. Each plays a vital role in ensuring the AI system's outputs are reliable and dependable, directly influencing our faith in the system.
However, operational trust extends beyond these technical facets. Compliance with laws and regulations, robust security measures, the humility of the AI system in recognizing its limitations, and robust governance structures are equally vital in fostering operational trust.
Moreover, ethical considerations are paramount. Transparency in AI operations, mitigating bias and promoting fairness, and respecting privacy rights are non-negotiable aspects of responsible AI deployment. These considerations ensure that the system aligns with societal values and expectations, thereby fostering trust.
Our case study with the healthcare provider demonstrated how these principles could coalesce in real-world scenarios, leading to successful outcomes through trusted AI.
At Sumo Analytics, we believe in and practice these principles. We consider them integral to our work and essential to delivering reliable, trustworthy AI solutions.
As we conclude, we invite you, our readers, to reflect on the AI systems you interact with or manage within your organizations. Consider the level of trust you place in them, and evaluate them against the principles discussed in this article.
The future of AI is indeed bright, but it is trust that will truly illuminate its path. By fostering trust in our AI systems, we can unlock their transformative potential and guide their integration into our organizations and our lives in a responsible, ethical, and beneficial manner.
Sumo Analytics, a leading data science and AI research agency, specializes in prediction science, offering transformative solutions that propel businesses into the future. With a dedicated team of skilled data scientists, machine learning engineers, and AI strategists, Sumo Analytics harnesses the power of advanced algorithms, robust data sets, and cutting-edge technology to predict trends, decipher complex patterns, and drive informed decision-making. Through our bespoke AI models, we not only provide accurate forecasts but also ensure transparency, fairness, and privacy, aligning with the highest ethical standards. We are deeply committed to fostering trust in AI, recognizing it as the cornerstone of successful AI integration. With Sumo Analytics, businesses can confidently navigate the complexities of the digital age, leveraging AI-driven insights to maximize efficiency, innovate processes, and ultimately, achieve sustainable growth.